V2V4Real
The first large-scale, real-world multimodal dataset
for Vehicle-to-Vehicle (V2V) perception
What does V2V4Real provide?
V2V4Real is the first large-scale real-world dataset for Vehicle-to-Vehicle (V2V) cooperative perception in autonomous driving.
It features:
- Collected by two vehicles simultaneously in the same location, providing multi-view sensor datastream
- 410 km of the driving area, 20K LiDAR, 40K RGB, and 240K annotated 3D bounding boxes across 5 vehicle classes.
- Diverse road types included: Intersections, highway entrance ramps, straight highway roads, and straight city roads.
- High-definition (HD) maps provided
- Three cooperative perception tasks supported: 3D object detection, object tracking, and Sim2Real domain adaptation, with benchmarks using sota models.
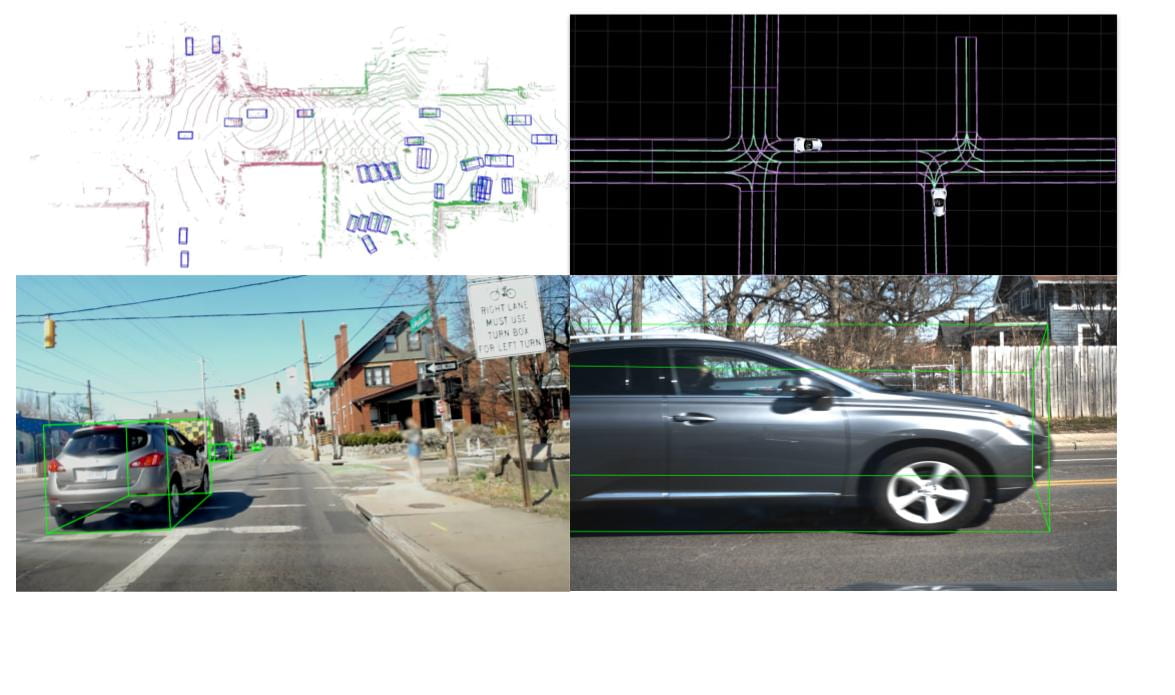
Data Collection
LiDAR:
- velodyne 32 channel lidar
- 1.2M points per second, 10 Hz
- 200m capturing range
- −25◦ to 15◦ vertical FOV , ±3 cm error
Camera:
- 2x RGB camera with 1920 × 1080 resolution, 110◦ FOV
Localization:
- Tesla: RT3000
- Ford: Novatel SPAN E1
Collection route:
- Freeway with one to five lanes
- City road, one to two lanes
- Highway, two to four lanes
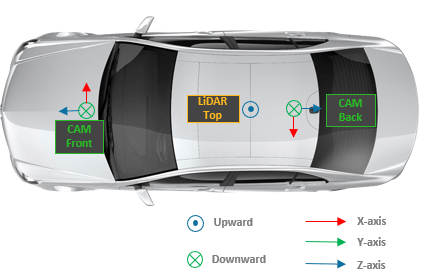
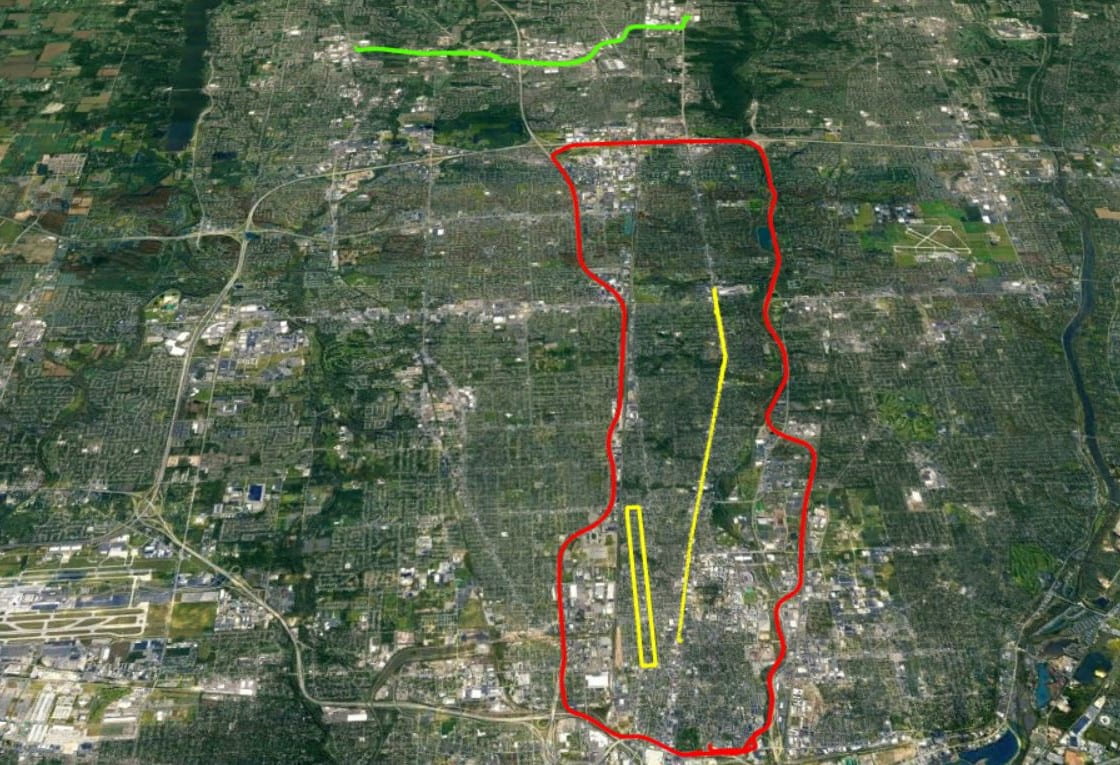
Data Annotation
3D Bounding Boxes Annotation:
- SusTechPoint is used to annotate 3D bounding boxes for LiDAR data.
- Two groups of professional annotators label and refine five object classes.
- Each object is annotated with its 7-degree-of-freedom 3D bounding box, driving state, and consistent ID and size.
- Consistent ID and size are assigned to the same object in different timestamps.
Map Annotation:
- HD map generation pipeline includes a global point cloud map and vector map.
- LiDAR odometry is constructed using the transformation and GPS/IMU information.
- Points are transformed onto the map coordinate to form a global point cloud map.
- OpenDRIVE maps are outputted and converted to lanelet maps as the final format.
Benchmark
Cooperative 3D object detection benchmark
Sync |
Async |
AM |
|||
Method |
AP@IoU=0.5 |
AP@IoU=0.7 |
AP@IoU=0.5 |
AP@IoU=0.7 |
(MB) |
No Fusion |
39.8 |
22.0 |
39.8 |
22.0 |
0 |
Late Fusion |
55.0 |
26.7 |
50.2 |
22.4 |
0.003 |
Early Fusion |
59.7 |
32.1 |
52.1 |
25.8 |
0.96 |
F-Cooper |
60.7 |
31.8 |
53.6 |
26.7 |
0.20 |
V2VNet |
64.5 |
34.3 |
56.4 |
28.5 |
0.20 |
AttFuse |
64.7 |
33.6 |
57.7 |
27.5 |
0.20 |
V2X-ViT |
64.9 |
36.9 |
55.9 |
29.3 |
0.20 |
CoBEVT |
66.5 |
36.0 |
58.6 |
29.7 |
0.20 |
Cooperative Tracking benchmark
Method |
AMOTA(↑) |
AMOTP(↑) |
sAMOTA(↑) |
MOTA(↑) |
MT(↑) |
ML(↓) |
No Fusion |
16.08 |
41.60 |
53.84 |
43.46 |
29.41 |
60.18 |
Late Fusion |
29.28 |
51.08 |
71.05 |
59.89 |
45.25 |
31.22 |
Early Fusion |
26.19 |
48.15 |
67.34 |
60.87 |
40.95 |
32.13 |
F-Cooper |
23.29 |
43.11 |
65.63 |
58.34 |
35.75 |
38.91 |
V2VNet |
28.64 |
50.48 |
73.21 |
63.03 |
46.38 |
28.05 |
AttFuse |
30.48 |
54.28 |
75.53 |
64.85 |
48.19 |
27.83 |
V2X-ViT |
30.85 |
54.32 |
74.01 |
64.82 |
45.93 |
26.47 |
CoBEVT |
32.12 |
55.61 |
77.65 |
63.75 |
47.29 |
30.32 |
Domain Adaptation benchmark
Method |
AP@IoU=0.5 |
AP drop |
AttFuse |
22.5 |
42.2 |
AttFuse w/D.A. |
23.4 (+0.9) |
41.3 |
F-Cooper |
23.6 |
37.1 |
F-Cooper w/D.A. |
37.3 (+13.7) |
23.4 |
V2VNet |
23.2 |
41.3 |
V2VNet w/D.A. |
26.3 (+3.1) |
38.2 |
V2X-ViT |
27.4 |
37.5 |
V2X-ViT w/D.A. |
39.5 (+12.1) |
25.4 |
CoBEVT |
32.6 |
33.9 |
CoBEVT w/D.A. |
40.2 (+7.6) |
26.3 |
Paper + Github
Download
LiDAR + Labels (OPV2V format)
All Rights Reserved
Contact Us: jiaqima@ucla.edu